This is a public peer review of Scott Alexander’s essay on ivermectin, of which this is the fourteenth part. You can find an index containing all the articles in this series here.
In our previous episode, we completed the analysis of all the studies Scott covered. After those, the essay continues with the logical next step: a review of the state of play—and a meta-analysis of sorts—of the studies he retained.
This essay will cover the entire section called “The Analysis,” so it’s going to be on the long side. Grab a beverage of your choice with one hand, hang on to your hats with the other, and let’s dive in.
Separating Chaff from Wheat
In the first part of this section, Scott summarizes his prior findings and his rationale for keeping certain studies:
The Analysis
If we remove all fraudulent and methodologically unsound studies from the table above, we end up with this:
Gideon Meyerowitz-Katz, who investigated many of the studies above for fraud, tried a similar exercise. I learned about his halfway through, couldn’t help seeing it briefly, but tried to avoid remembering it or using it when generating mine (also, I did take the result of his fraud investigations into account), so they should be considered not quite independent efforts. His looks like this:
He nixed Chowdhury, Babaloba, Ghauri, Faisal, and Aref, but kept Szenta Fonseca, Biber (?), and Mayer. There was correlation of 0.45, which I guess is okay.
A good reminder that even Gideon Meyerowitz-Katz does not consider Biber and Mayer exclusion-worthy, even though—especially in the case of Biber—Scott’s exclusion is based on GidMK’s erroneous insinuations of p-hacking.
I asked him about his decision-making, and he listed a combination of serious statistical errors and small red flags adding up. I was pretty uncomfortable with most of these studies myself, so I will err on the side of severity, and remove all studies that either I or Meyerowitz-Katz disliked.
Remember this part: that Scott is “erring on the side of severity” here. It will become relevant down the line.
We end up with the following short list:
We’ve gone from 29 studies to 11, getting rid of 18 along the way. For the record, we eliminated 2/19 for fraud, 1/19 for severe preregistration violations, 10 for methodological problems, and 6 because Meyerowitz-Katz was suspicious of them.
…but honestly this table still looks pretty good for ivermectin, doesn’t it? Still lots of big green boxes.
Meyerowitz-Katz accuses ivmmeta of cherry-picking what statistic to use for their forest plot. That is, if a study measures ten outcomes, they sometimes take the most pro-ivermectin outcome.
This is something that an honest evaluator would document with examples. I am not aware of any such illustrations. Come to think of it, in personal communication, Dr. Avi Bitterman mentioned that he found an issue with the endpoint used in Ghauri et al.—which he told ivmmeta about—and they promptly corrected it. More evidence that ivmmeta are willing to accept feedback even by those who publicly attack them, and that they take their protocol very seriously. Scott’s claim that they are using the wrong endpoints without making even a basic attempt to document what those are feels extremely unfair, if only because we can’t actually evaluate its veracity.
I suppose this is where we apply Hitchens’ razor and dismiss without evidence what is offered without evidence.
Ivmmeta.com counters that they used a consistent and reasonable (if complicated) process for choosing their outcome of focus, that being:
If studies report multiple kinds of effects then the most serious outcome is used in calculations for that study. For example, if effects for mortality and cases are both reported, the effect for mortality is used, this may be different to the effect that a study focused on. If symptomatic results are reported at multiple times, we used the latest time, for example if mortality results are provided at 14 days and 28 days, the results at 28 days are used. Mortality alone is preferred over combined outcomes. Outcomes with zero events in both arms were not used (the next most serious outcome is used — no studies were excluded). For example, in low-risk populations with no mortality, a reduction in mortality with treatment is not possible, however a reduction in hospitalization, for example, is still valuable. Clinical outcome is considered more important than PCR testing status. When basically all patients recover in both treatment and control groups, preference for viral clearance and recovery is given to results mid-recovery where available (after most or all patients have recovered there is no room for an effective treatment to do better). If only individual symptom data is available, the most serious symptom has priority, for example difficulty breathing or low SpO2 is more important than cough.
I’m having trouble judging this, partly because Meyerowitz-Katz says ivmmeta has corrected some earlier mistakes, and partly because there really is some reasonable debate over how to judge studies with lots of complicated endpoints.
Wait, is the fact that they have corrected earlier mistakes a claim against their credibility? I’m not sure I can interpret what Scott writes here differently. Also keep in mind that ivmmeta has used this protocol since inception, and in fact this protocol was used in hcqmeta.com when it launched, back in October 2020. This same protocol is used in all 40+ websites included in c19early.com, so any concern that it was tailor-made for ivermectin must account for that fact and promptly self-immolate.
Meta-analytical Shenanigans
Let’s get to the most important part. In my humble opinion, this is where the entire essay collapses. But don’t take my word for it, follow along and see for yourself.
First, let’s read what he writes without interruption:
By this point I had completely forgotten what ivmmeta did, so I independently coded all 11 remaining studies following something in between my best understanding of their procedure and what I considered common sense. The only exception was that when the most severe outcome was measured in something other than patients (ie average number of virus copies per patient), I defaulted to one that was measured in patients instead, to keep everything with the same denominator. My results mostly matched ivmmeta’s, with one or two exceptions that I think are within the scope of argument or related to my minor deviations from their protocol.
Probably I’m forgetting some reason I can’t just do simple summary statistics to this, but whatever. It is p = 0.15, not significant.
This is maybe unfair, because there aren’t a lot of deaths in the sample, so by focusing on death rather than more common outcomes we’re pointlessly throwing away sample size. What happens if I unprincipledly pick whatever I think the most reasonable outcome to use from each study is? I’ve chosen “most reasonable” as a balance between “is the most severe” and “has a lot of data points”:
Now it’s p = 0.04, seemingly significant, but I had to make some unprincipled decisions to get there. I don’t think I specifically replaced negative findings with positive ones, but I can’t prove that even to myself, let alone to you.
Scott is claiming to do a meta-analysis, producing results which he evaluates for statistical significance. Given the severity with which he has approached scientists so far, you’d expect him to do this in a principled and disciplined manner that is defensible against any claims of sloppiness or bias.
Right?
…right?
Reverse Engineering Scott’s Analysis
Before we go further, let’s figure out how Scott got his numbers. What you see here is the exact formula that replicates his results, known as a paired sample t-test. We can be pretty sure this is what he did—not just because the numbers match up precisely—but also because he hints at it later in the essay:
In this very essay, I almost said that a set of ivermectin studies showed a positive result because I was reading the number for whether two lists were correlated rather than whether a paired-samples t-test on the lists was significant.
So here are what the results look like in my replication:
As you can see, a simple Excel/Google Sheets formula is enough to get us the same numbers Scott got.
Bad Level 1 - Wrong Event Numbers
Here’s the thing: once we look at the actual numbers, we notice some issues— particularly in the second analysis where Scott did his own data extraction rather than relying on ivmmeta. Scott hasn’t represented the numbers fairly and/or accurately in three cases:
Neither ivmmeta or I can figure out where the Mahmud data is from, and they look significantly worse than the data from the paper itself.
For Krolewiecki, the numbers don’t correspond to any of the actual outcomes. Scott may have scaled them to account for different group sizes. However, by rounding them into integers, he has distorted the results.
In the case of Lopez-Medina, Scott chose a post-hoc exclusion endpoint that removes four events from the control group.
So here’s what the analysis looks like once we correct these three issues (corrections in red):
Interestingly, we see that the “significant” second result gets more significant than Scott showed it to be. But don’t take this result to the bank yet, we’re not done fixing things.
Bad Level 2 - Ignoring Patient Numbers
You see, while Scott tries to balance numbers—specifically in the case of Krolewiecki—he ignores the problem of imbalanced numbers across studies in general. In fact, he doesn’t take patient numbers into account at all.
For example, if a study had 10 events in 20 patients in each arm, as far as Scott’s analysis is concerned, that would be the same as having 10 events in 2000 patients, since there’s no accounting for patient numbers. Of course, that makes no sense, but we can fix that by bringing patient populations back, and comparing percentages rather than event numbers:
Holy smokes! The results are completely different. Now, ivermectin doesn’t look like it has a statistically significant result at all! For the analysis using ivmmeta endpoints, we get a p-value of 0.25, whereas for the analysis with Scott’s preferred endpoints we get p=0.06.
Does that mean his original conclusion was actually correct?
Not even close, I’m afraid.
The obvious issue here is that to produce such an analysis, we must consider each study to be equal to every other study, regardless of the number of patients enrolled. That can’t possibly be right. There must be a way to weigh each study differently, right? Indeed. In fact, you will not be surprised to know that the field of meta-analysis has spent substantial effort on this question—effort which Scott has ignored—since he is not using the right tool for the job he’s attempting to do.
Bad Level 3 - The Wrong Algorithm
Now, some of you have read my piece on Scott’s use of the t-test, are aware of the ensuing drama, and know where this is going. For the rest of you, here’s a recap:
The very formula Scott uses—the paired sample t-test—is wildly inappropriate. Actually, even to call it inappropriate is giving it too much credit. The test Scott used has no business being in the same region of concept-space as a meta-analysis, never mind being used to draw conclusions.
To break it down to its simplest possible form, here’s Scott’s original analyses:
And here’s what happen if we add an extra study that is wildly pro-ivermectin:
Notice that the p-values go UP (i.e. the effect now looks less significant) while a meta-analysis with a seemingly positive study added should end up with the effect looking *more* significant (i.e. smaller p-value). In fact, the second analysis (on the right) goes from being statistically significant to being statistically not significant.
Might as well decide the result of the meta-analysis by throwing dice.
What should we use instead? You see, when doing a meta-analysis, there’s a lot of academic debate about what formula to use. The standard approach most people use is the DerSimonian and Laird approach. From Tess Lawrie’s team to Gideon Meyerowitz-Katz (and again) to Cochrane to Andrew Hill to Ivmmeta. As the Wikipedia article on meta-analysis puts it:
The most widely used method to estimate between studies variance (REVC) is the DerSimonian-Laird (DL) approach.[43] Several advanced iterative (and computationally expensive) techniques for computing the between studies variance exist (such as maximum likelihood, profile likelihood and restricted maximum likelihood methods)
However, a comparison between these advanced methods and the DL method of computing the between studies variance demonstrated that there is little to gain and DL is quite adequate in most scenarios.[47][48]
There are other approaches, and there are also questions about what the limits of applicability of DerSimonian-Laird are. Obviously, if I had my choice of methods, I’d go with a Bayesian approach, like Neil and Fenton did here. However, the one thing that is certain is that t-tests—as Scott used them—are not even in the conversation. It is as basic an error as it gets. As you can see above, he didn’t even apply those properly, in part because it’s not really obvious how to apply them properly.
You might ask if all this formula talk makes a difference. Let’s actually look at the comparison.
When re-doing Scott’s first analysis using using Cochrane’s RevMan (which appropriately applies DerSimonian-Laird here) I get the following result:
Instead of Scott’s p=0.15 (which we corrected to p=0.25), RevMan actually gives us p=0.03, which is a statistically significant positive result for ivermectin, indicating 55% improvement.
This is despite removing everything Scott and Gideon removed, including all the studies they misread, etc.
When I informed the people at ivmmeta of the issue—to cross-check my thinking—they responded by posting a DerSimonian-Laird replication of Scott’s second meta-analysis:
They even left Scott’s errors in copying numbers in there. Regardless, they found that instead of p=0.04, he should have gotten p=0.0046, a strongly statistically significant result.
You’re reading this right. Scott went through thousands of words, and dragged so many scientists through the mud for not being thorough and not following proper procedures to a tee. Not only was he dead wrong in many cases, but he himself performed one of the worst meta-analyses in history of meta-analyses. This isn’t exaggeration. If your analysis throws away something on the order of 90% of the evidence you gathered, the result has nothing to do with the underlying reality. As a way to think about this, consider that no drug would ever get approved if we used Scott’s approach to meta-analysis. That dog simply won’t hunt.
Not only that, but of the thousands and thousands of people who read and shared his work, nobody even noticed this most basic of errors.
Bad Level 4 - Ignoring Corrections
Now, you might imagine that upon being told of an oversight of this magnitude, Scott would go back and revise his conclusions.
Not so much. After an email back-and-forth that took about a month, I got him to acknowledge the issue in the article, so that’s the good part:
[UPDATE 5/31/22: A reader writes in to tell me that the t-test I used above is overly simplistic. A Dersimonian-Laird test is more appropriate for meta-analysis, and would have given 0.03 and 0.005 on the first and second analysis, where I got 0.15 and 0.04. This significantly strengthens the apparent benefit of ivermectin from ‘debatable’ to ‘clear’. I discuss some reasons below why I am not convinced by this apparent benefit.]
As you can see, his response is to say that the t-test is “overly simplistic”. The only universe in which a t-test could be called “overly simplistic” is one where we also consider a coin-toss to be an “overly simplistic” method of diagnosing lung cancer.
Further, he says that replacing his evidence-shredding approach with the standard meta-analysis approach doesn’t change his conclusions. He did not retract his article, nor did he alert his readers to the correction, other than to update his mistakes page.
Sit back and meditate on this for a minute. We’ve gone about 2/3 into Scott’s essay— which so far has been dedicated to weeding out studies so he can do a meta-analysis that showed a “debatable”—in Scott’s words—benefit. When shown that he made an error that threw away something like 9/10 of the evidentiary strength of the studies he himself handpicked—even after “erring on the side of severity”—his response is that he is “not convinced by this apparent benefit” that he himself uncovered. This result is not produced by anything I decided here, other than correcting the algorithm Scott used to combine the studies he chose, which Scott recognized was a better choice.
Let’s continue working through the essay to see why this, while understandable on a psychological level, is the most unscientific possible reaction to being shown an error that is impactful on that kind of scale.
As with so many things, the issue is almost never the original error. It’s the response to being shown the error that is most revelatory.
Bad Level 5 - Projecting Onto Ivmmeta
As we continue through the essay, we see Scott juxtaposing his own results that are on the “edge of significance” with ivmmeta’s results:
(how come I’m finding a bunch of things on the edge of significance, but the original ivmmeta site found a lot of extremely significant things? Because they combined ratios, such that “one death in placebo, zero in ivermectin” looked like a nigh-infinite benefit for ivermectin, whereas I’m combining raw numbers. Possibly my way is statistically illegitimate for some reason, but I’m just trying to get a rough estimate of how convinced to be)
Even after being told that his results were simply the result of tragic methodological confusion on his part, he left the rest of the essay untouched, which means this is still there. Scott attempts to make some complex methodological point—that of course is not the reason for the difference in results. The reason his conclusions are less strong than those of ivmmeta is that:
He excluded many of the studies that ivmmeta used, several for reasons that do not correspond with reality
He used bad math to come to his conclusions on the remaining studies
To know that, and still have the gall to leave paragraphs such as this one in the essay, right below the text of the correction is simply beyond me. Obviously, Scott did not do this consciously. He simply did not care enough to review the essay and correct the commentary that is built upon his erroneous analysis.
Bad Level 6 - Warped Counsel
If you’ve read A Conflict Of Blurred Visions, the rest of this section will be familiar to you. Feel free to skip it. For everyone else—or perhaps for those who would like a refresher—read on:
Having corrected the choice of formula, we now turn our attention to the exclusions Scott made, “erring on the side of severity.” While Scott’s original exclusions look somewhat understandable (though much less so than I initially thought), the five papers that Scott excluded because of Gideon Meyerowitz-Katz, look to be either big and positive studies, or small and very positive studies.
Back when I first read Scott’s article—and being highly suspicious that these exclusions by GidMK had shifted the analysis entirely, but not wanting to let that prevent me from seeing something that was true—I decided to tie myself to the mast. I actually declared my intention to do a triple exclusion analysis, to see what the studies accepted by Scott Alexander, Gideon Meyerowitz-Katz, and ivmmeta.com show, as a consensus set of three opinions that come from very different places.

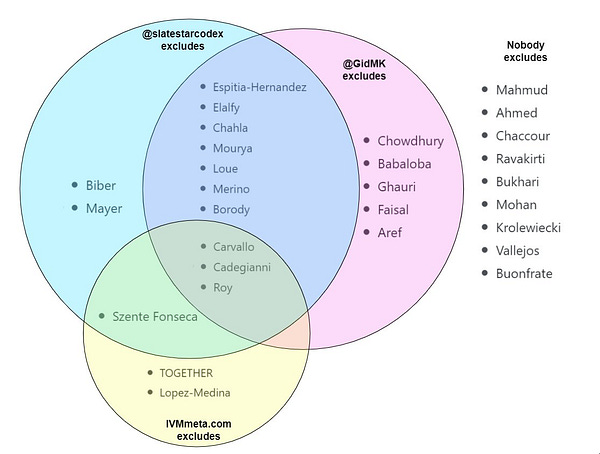
The next morning, I added more details about the experiment I was intending to run. I wanted to see what Scott’s exclusions would do on their own, what GidMK’s exclusions did when added to Scott’s exclusions, and what the ivmmeta.com exclusions would do when added to Scott’s exclusions—as well as when added to Scott’s and GidMK’s exclusions. That last one was the originally promised “triple exclusion” analysis. In short, I wanted to see these five analyses:
I pulled some favors, and I have fascinating results to share.
To start, here’s the complete set of early treatment ivermectin studies as presented at ivmmeta.com, coming to roughly the same result. Early treatment with ivermectin produces 67% improvement, or, as RevMan outputs it, has a risk ratio (RR) of 0.33 with a 95% confidence interval (95% CI) of 0.23-0.47:
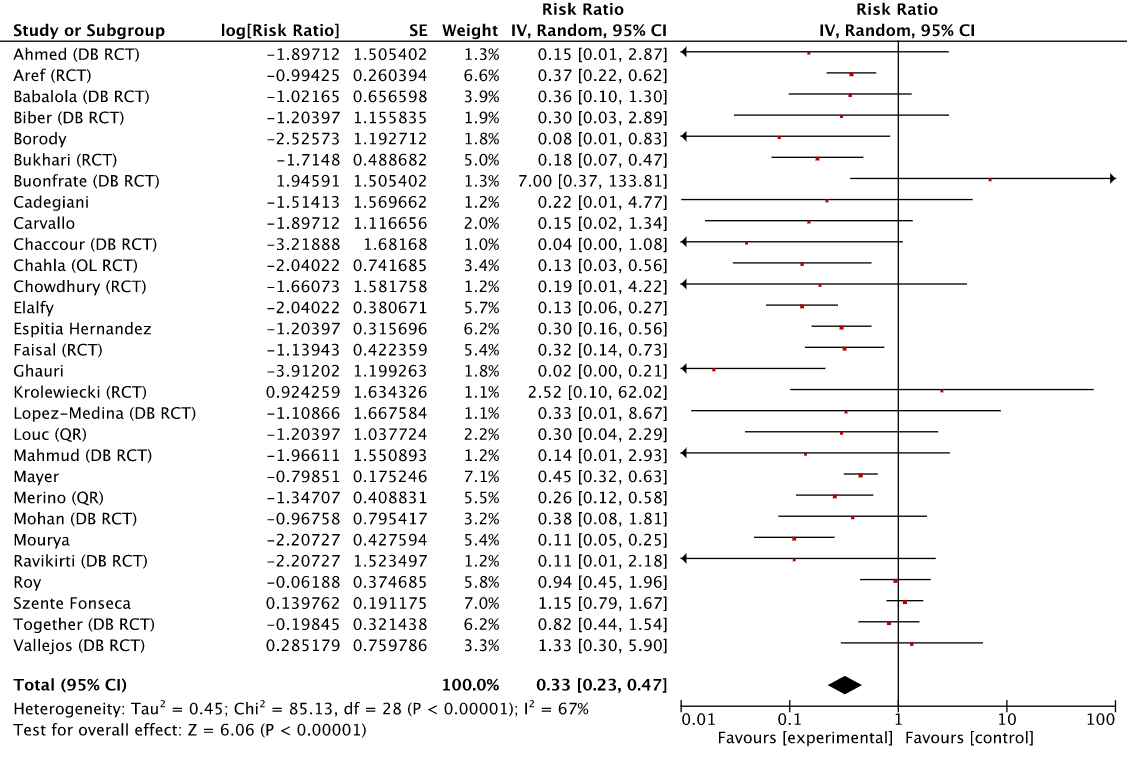
We then move on to the set of studies after Scott’s exclusions. Notice that the risk ratio (RR) barely moves (0.36 or 64% improvement), though the 95% confidence interval widens, if only a bit.
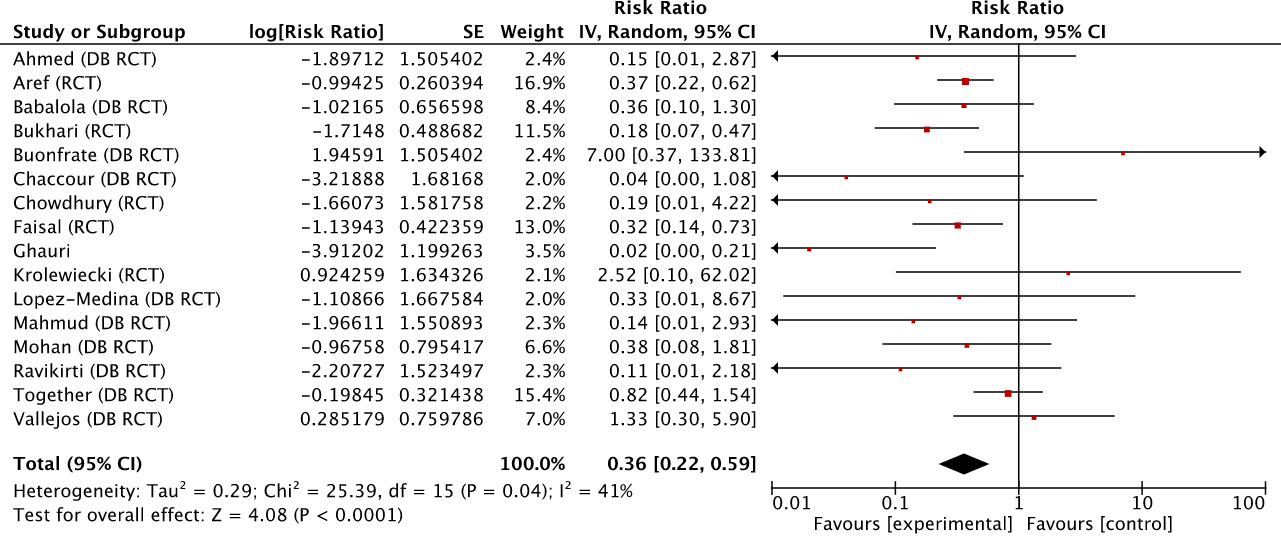
For brevity, I’ll spare you the third analysis which I will summarize below, and move on to the fourth. Let’s see what happens when we remove the studies recommended for exclusion by Gideon Meyerowitz-Katz.
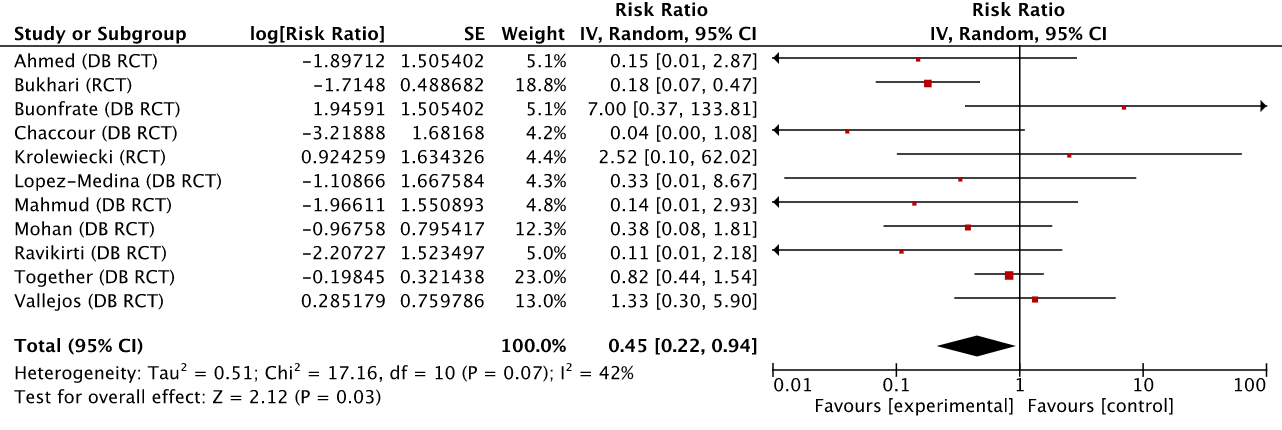
The difference is far larger than the one caused by Scott Alexander’s exclusions. The risk ratio moves to 0.45 (or 55% improvement). About 9% of the effect of ivermectin evaporates—and more importantly—the lower bound of the 95% confidence interval is stretched all the way to 0.94. For those of you into P-values (which don’t mean what the vast majority think they mean), we go from P<0.0001 without GidMK’s exclusions to P<0.03 with them. In other words, the exclusions recommended only by GidMK make the analysis on the remaining set of studies far more uncertain, though it does stay firmly within what is considered “statistically significant” territory. I’m not sure how Scott ended up with a P<0.15 but the difference is large enough to make me suspect a mathematical error somewhere.
If, in addition, we remove the two studies in this set that ivmmeta.com excludes (Lopez-Medina and TOGETHER), we are left with the originally promised triple exclusion analysis. The remaining studies are accepted by Scott Alexander, Gideon Meyerowitz-Katz, and ivmmeta.com.
The resulting Risk Ratio is 0.38 (or 62% improvement), somewhere between the one we started with, and what it was after the GidMK exclusions. While the 95% CI has widened, it is remarkable that we are still within the bounds of “statistical significance.” Not because that is the be-all and end-all of tests, but because we get to avoid a pointless argument on the meaning of p-values and the bright line fallacy.
Overall, the five pre-announced analyses put together end up looking something like this:
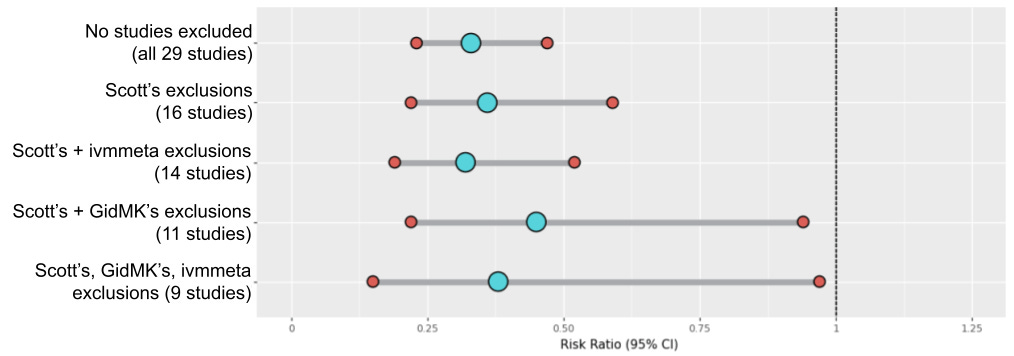
The way to read this chart, in brief, is that smaller intervals are better, and being more to the left is better. The Risk Ratio, very briefly, is how much better a patient taking the intervention (in this case ivermectin) does at whatever the relevant objective is than the control group. So a RR of 0.33 indicates that the intervention group does 3x better than the control group. ivmmeta.com chose the most serious endpoint in each study, which I find to be a pragmatic compromise for the kind of bottom-up dataset we’re looking at.
It is incredibly clear that Scott’s own analysis doesn’t actually move the conclusion much from what ivmmeta.com shows, even though he excluded almost half the studies. His trust in GidMK waters down the power of the dataset to the point where the effect starts to look uncertain, even if ivermectin still looks more likely than not to have a significant effect.
When you torture a dataset this much, and it still doesn’t tell you what you want to hear, well, you have to start wondering if maybe the poor dataset really believes what it’s telling you. I must admit that I did not expect that after all the exclusions we would still be in commonly accepted as “significant” territory, but them’s the maths.
Nevertheless, none of this should be taken to be an official meta-analysis, or even particularly scientific. What I intended to show—and I think this exercise makes clear—is that Scott’s conclusions are very much dominated by what he excluded because of Gideon Meyerowitz-Katz. Had he not done so, the conclusion of his analysis would have been very different. After all, this is the person that posted this Tweet way back before the vast majority of studies were completed—or, for some—even started:
Bad Level 7 - Rhetorical Manipulation
So how does the essay proceed? Given that it is uncorrected from the time before he knew that his results were in error, Scott proceeds to take the results he originally got, and use rhetorical techniques to make them look even more significant.
So we are stuck somewhere between “nonsignificant trend in favor” and “maybe-significant trend in favor, after throwing out some best practices”.
First, he waters down the result by calling it “maybe significant” and only “after throwing out some best practices.” The throwing out of best practices was, of course, Scott’s doing. When he did that he got “definitely statistically significant effect.” So in light of his erroneous results to begin with, this sentence is already muddying the water. A precise description would be “statistically significant effect after throwing out some best practices.”
This is normally where I would compare my results to those of other meta-analyses made by real professionals. But when I look at them, they all include studies later found to be fake, like Elgazzar, and unsurprisingly come up with wildly positive conclusions. There are about six in this category. One of them later revised their results to exclude Elgazzar and still found strong efficacy for ivermectin, but they still included Niaee and some other dubious studies.
The only meta-analysis that doesn’t make these mistakes is Popp (a Cochrane review), which is from before Elgazzar was found to be fraudulent, but coincidentally excludes it for other reasons. It also excludes a lot of good studies like Mahmud and Ravakirti because they give patients other things like HCQ and azithromycin - I chose to include them, because I don’t think they either work or have especially bad side effects, so they’re basically placebo - but Cochrane is always harsh like this. They end up with a point estimate where ivermectin cuts mortality by 40% - but say the confidence intervals are too wide to draw any conclusion.
These two paragraphs need so much examination that instead of trying to cover the convoluted matter of meta-analyses here, I will probably cover it as a separate article. Scott gets some important things right here, but is also unaware of extremely important information and ultimately buries the lede. Until then, see my piece on how the Cochrane meta-analysis by Popp et al. violated all its own criteria to include some studies while excluding others.
I think this basically agrees with my analyses above - the trends really are in ivermectin’s favor, but once you eliminate all the questionable studies there are too few studies left to have enough statistical power to reach significance.
This isn’t what he found though, especially after correcting for the use of the t-test.
Except that everyone is still focusing on deaths and hospitalizations just because they’re flashy. Mahmud et al, which everyone agrees is a great study, found that ivermectin decreased days until clinical recovery, p = 0.003?
So what do you do?
This is one of the toughest questions in medicine. It comes up again and again. You have some drug. You read some studies. Again and again, more people are surviving (or avoiding complications) when they get the drug. It’s a pattern strong enough to common-sensically notice. But there isn’t an undeniable, unbreachable fortress of evidence.
I hope you’ve noticed that Scott is—once again—making assertions that do not follow from his own analysis.
The drug is really safe and doesn’t have a lot of side effects. So do you give it to your patients? Do you take it yourself?
Here this question is especially tough, because, uh, if you say anything in favor of ivermectin you will be cast out of civilization and thrown into the circle of social hell reserved for Klan members and 1/6 insurrectionists. All the health officials in the world will shout “horse dewormer!” at you and compare you to Josef Mengele. But good doctors aren’t supposed to care about such things. Your only goal is to save your patient. Nothing else matters.
This might be one of the most remarkable paragraphs in his whole piece. Scott understands that there is immense social pressure to find ivermectin does not work. If we’re being strict about it, any evidence that emerged after this Tweet should be considered tainted, given that this is a pretty direct threat to the career of any perceptive scientist who saw it:
Can you name another time a US regulator took such a public stance against a drug that was being actively investigated at the time? I sure haven’t heard of any such case. The FDA even doubled down a few months ago:

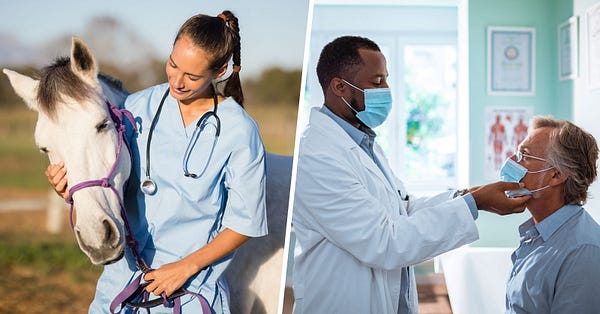
Are we seriously telling ourselves that after such a public position they would review the evidence fairly and approve the drug for COVID-19 if the studies returned a strong positive conclusion?
And yet, Scott—knowing all this and much more—does not use this information to temper his analysis. I’m also pretty sure he did not seek out any ivermectin proponents while writing his piece. I was in conversation about this topic with some of his close friends, so if had he asked around, he would have been pointed my way by multiple people.
Instead, he put out this essay, which simply did more of the same—it amplified the cycle of vilification and humiliation of the scientists who studied this drug and reported their findings as best as they could.
Scott completes the analysis section thus:
I am telling you that Mahmud et al is a good study and it got p = 0.003 in favor of ivermectin. You can take the blue pill, and stay a decent respectable member of society. Or you can take the horse dewormer pill, and see where you end up.
In a second, I’ll tell you my answer. But you won’t always have me to answer questions like this, and it might be morally edifying to observe your thought process in situations like this. So take a second, and meet me on the other side of the next section heading.
…
…
…
…
…
Now, if you’ve read his essay, you know where all this is going, but I will stop here for now, and continue with the next section in a future installment of this series.
This is a public peer review of Scott Alexander’s essay on ivermectin, of which this is the thirteenth part. You can find an index containing all the articles in this series here.
While I don't consider the target of your critique to be a scientist, his biased mindset and dishonest methods are far from uncommon among academic scientists. A couple of anecdotes from my 30 years in academic biology.
Shortly after I retired, I was eating at a crowded cafe next to the university that generously gave me a doctorate. At the next table were a middle-aged man and a young man, the former apparently being the graduate advisor and the latter his grad student. The conversation consisted of the advisor recounting with evident pride how he had blocked an academic rival's publication and his participation in a symposium. The man was as physically repulsive as morally, and I easily imagined that his only pleasure in life was dirty dealing.
As an evolutionary ecologist, I often took up projects in subareas that were new to me. After all, evolution intersecting with ecology spans a huge range of phenomena. Usually in publishing I had some trouble with territorial reviewers, but always managed to satisfy editors. The worst reviewer corruption, however, was appalling. That fellow's review stated that if he were put on as an author and rewrote one section, then the paper could be accepted for publication.
An editor of a journal bragged to a group of grad students that he had rejected a manuscript because it used a one-sided t-test instead of a two-sided. Rejected, not requested a recalculation and resubmission of the manuscript.
There are plenty of people in science who, while as pacific as can be, display attitudes that would be attributed to anti-social personality disorder if they were physical acts rather than attitudes and tricks of the trade.
Thank you for all this great work. I just commented over at Scott's substack, urging him to read this and correct his article.