The good thing about sampling many disciplines, is that sometimes you see the same principle emerge—with a different name, slant, and emphasis—but building that Rosetta stone then allows you to transfer insights across, and/or spot outlier disciplines who seem to dissent.
Here’s one thing I’ve noticed doing that over the last decade or two: when systems (organizations, life forms, algorithms...) have a life-or-death need to align to reality as precisely as possible, they tend to combine heterogeneous types of evidence to arrive at conclusions.
Here are all the examples I’ve been able to gather of what I’m talking about:
Robotics
The first example of the concept in question is closer to my home turf: robotics has the concept of Sensor Fusion, a technique for merging different types of sensor data to map an environment.
The idea is that since the sensors are built differently, and often interact with reality using distinct channels, the only correlation should be reality itself. No technical implementation issues should interfere, since no technical implementation is shared.
Hedge Funds
In a different context, hedge funds are famous for using any dataset they can get their hands on to predict a company's financial results. The use of "alternative data" is now seen more often than not.
This practice hails from the most legendary hedge fund of all time, Renaissance Technologies, founded by mathematician Jim Simmons. They started by combining data nobody else had, and they’ve kept running since.
Ev Psych
So, we have robots, hedge funds and... evolutionary psychology?
"nomological networks of cumulative evidence stimulate greater interdisciplinarity, lesser methodological myopia, and increased consilience (unity of knowledge)."
Probably a good place to append @jordanbpeterson’s methodological triangulation:

Astronomy
Where else? How about the universe?
"Multi-messenger astronomy is the practice of synthesizing these various messengers from violent astronomical events."
This is the other kind of MMA... but also about violent events with galaxy-shattering consequences.
Data Science
Trying to remember where I first came across this concept, my mind goes to the Netflix challenge, which ended up dominated by ensemble networks: many models, some of them trained on other models, orchestrated to work as one, to make better predictions.
Biology
And as @oldbooksguy highlighted on Twitter, the body (surprise!) works in a very similar way.
"...how information from the different sensory modalities (such as sight, sound, touch, smell, self-motion, and taste) may be integrated by the nervous system…"
In a strange turn, looking into an upstream robotics technique called simultaneous localization and mapping (SLAM), brought me right back to the brain—and in particular the hippocampus—that seems to operate similarly.
By the way, you might not need the different kinds of sensors to benefit. Your brain uses parallax to combine your eyes' 2D vision into something far more valuable—3D vision.
In fact, you might not even need multiple eyes to get the benefit. If you're a pigeon.
Self-driving
I learned about this from @karpathy when presenting his work on Tesla's self-driving. Which, while using pure vision, takes advantage of motion as well as regular parallax to the extreme:
Journalism & Law
In less formal domains such as journalism and law, the concept of corroborating evidence comes up. Learning something from multiple sources—as unrelated as possible—should increase our confidence that it happened.
Can We Use This For Fun and Profit?
Well everyone else is, so I don’t see why not.
For me, this idea of multi-modal integration provides an answer to a problem that's been bugging me for a while: how do we identify true signal in a world of information warfare, where everything that can be faked, will be faked.
This is the idea behind drug cocktails: instead of narrowly identifying and disrupting a pathogen with a monotherapy it can easily evolve around, much better to identify and disrupt multiple properties at once, not giving any room to evolve away from the combined attack with its basic functions intact.
So What About Medicine?
In comparison to all the domains we've seen so far—where heterogeneous signals are the gold standard of reality coupling—modern medicine, often humbly known as Evidence-Based Medicine, appears to put most of its eggs in one basket: The large-scale Randomized Controlled Trial (RCT).
Knowing all this, what would we expect from the dependence on RCTs? Extremely high costs, slowness, and being subject to the quirks of the tool, to name a few things. Even if limited to speed and cost, only the largest companies can support such trials, and only for the most profitable treatments.
In Closing
If you've read this far, I have two questions:
Are you aware of other paradigms used in other disciplines that fit the pattern described here?
Does the abstract pattern itself have a name? Alternatively, has anyone else tried to catalogue all such similar patterns?
Before I go, here are some responses to the original thread this article came from that made me stop and think:
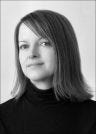
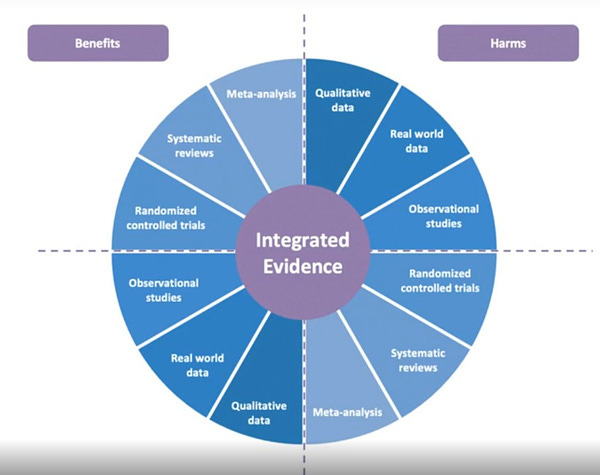
My God. Every ducking link in this blog post. Every ducking link.
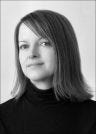
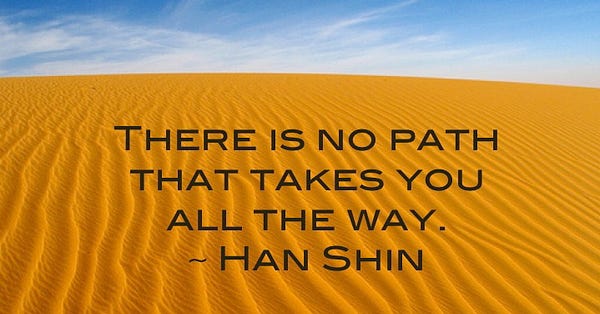
P.S. anyone notice that this article itself is an application of the concept it describes on the concept it describes?
Judeas Pearl is a famous researcher in AI. He invented a causal reasoning technique that I think would be extremely useful with the large dataset that ivmmeta.com provides. It is based on Bayes but includes causality with a ‘do’ operator. He dislikes RCTs and shows many examples where it would be possible to get a lot higher confidence for a fraction of the cost. I must admit that I started to read ‘the book of why’ several times and haven’t finished it yet, getting a good intuition around Bayes seems difficult for me. However, I think he is on to something. I think he would be right up your sleeve.
"Data assimilation" is (mainly) the terminology used for (roughly) this in weather forecasting. All sorts of space-time measurements being injected into Navier Stokes around the clock to continuously maintain initial conditions for simulations into the future. Those systems are pretty impressive.