This is a public peer review of Scott Alexander’s essay on ivermectin, of which this is the ninth part. You can find an index containing all the articles in this series here.
In today’s episode, we look into a theme that runs throughout Scott’s essay: his deference to randomized controlled trials (RCTs) above all other forms of evidence—in particular, how this bias affects his treatment of three non-RCT studies: Merino et al., Mayer et al., and Chahla et al.
Merino et al.
Let’s see what Scott has to say about this one:
Merino et al: Another (sigh) non-RCT. Mexico City tried a public health program where if you called a hotline and said you had COVID, they sent you an emergency kit with various useful supplies.
That is not what the study says. Patients who received a positive test for a test were *given* a kit (under certain conditions). Scott seems to be confusing kit distribution with the phone *monitoring* that a subset of the patients were offered. They were called a few days after they had received a positive test, to check how they felt and to advise them to go to hospital according to the description they gave of their own health state.
One of those supplies was ivermectin tablets. 18,074 people got the kit (and presumably some appreciable fraction took the ivermectin, though there’s no way to prove that).
In fact, 83,000 patients got the kit, out of whom 77,381 were included in the study. Of those, 18,074 received a monitoring phone call.
Their control group is people from before they started giving out the kits, people from after they stopped giving out the kits, and people who didn’t want the kits.
To my knowledge, Mexico City didn't stop giving out the kits (not right after the study, at least). Here's what the study mentions:
The control group are positive symptomatic patients, from 23 November to 28 December, and the treated group are positive symptomatic patients from 28 December to 28 January.
Let’s keep going:
There are differences in who got COVID early in the epidemic vs. later, and in people who did opt for medical kits vs. didn’t.
It doesn’t seem that the medical kit was opt-in. The paper says that the treatment group assumes “all cases with a positive antigen test and symptoms received the medical kit after the program began”. Obviously this would bias the results against the treatment, since it’s unclear how many of those patients actually got the kit, and how many actually used it.
Also, unlike Scott is suggesting, there is no "early in the pandemic" involved in the study: the time difference between control group and treated group is exactly 1 month: Nov 23-Dec 28 2020 for the control group, and Dec 28 2020-Jan 28 2021 for the treated group.
[My thanks to Enzo for pointing out the factual issues with Scott’s writeup of Merino et al.]
To correct these, the researchers tried to adjust for confounders, something which - as I keep trying to hammer home again and again - never works.
This is something Scott brings up again and again: observational trials are useless, only RCTs will do. He does link to a study to support his point: it's about how new and complex psychometrics have a hard time getting established over old and simple psychometrics. The math in the paper is complex, but the authors make it clear that their argument focuses on psychological domains and specifically around the issue of measurement uncertainty:
Social scientists often seek to demonstrate that a construct has incremental validity over and above other related constructs. However, these claims are typically supported by measurement-level models that fail to consider the effects of measurement (un)reliability. We use intuitive examples, Monte Carlo simulations, and a novel analytical framework to demonstrate that common strategies for establishing incremental construct validity using multiple regression analysis exhibit extremely high Type I error rates under parameter regimes common in many psychological domains.
I suspect the measurement uncertainty of psychological domains is far far higher than that involved in being hospitalized or dying of COVID-19. What I do know, is that the authors of the paper make sure to declare the scope of their argument very carefully, and—crucially—close their paper with a method of how to actually fix the issue they’re highlighting. This leaves Scott’s claim that such a thing “never works” unsupported by the very reference he’s using to support it.
Back to square one. Is there anything we can look at to decide this question?
Actually, yes! We can look at real-world data. Not only have people done many meta-analyses on this question, there’s a Cochrane meta-analysis of such meta-analyses, that seeks to answer once and for all if observational trials result in significantly different results than RCTs done against the same hypothesis.
Their answer? No.
Our results provide little evidence for significant effect estimate differences between observational studies and RCTs, regardless of specific observational study design, heterogeneity, inclusion of pharmacological studies, or use of propensity score adjustment. Factors other than study design per se need to be considered when exploring reasons for a lack of agreement between results of RCTs and observational studies.
Many studies I’ve found on the question seem to end up in the same place: observational trials and RCTs agree more often than not. Including observational trials in meta-analyses increases statistical power and helps with causal inference. When the two disagree, the causes should be sought in specific design decisions of each trial—not in the difference between types of trial—which doesn’t seem to be predictive.
No less than former FDA head Thomas Frieden wrote a paper in 2017 on this topic: Evidence for Health Decision Making—Beyond Randomized, Controlled Trials. He goes through a number of case studies and different types of evidence that motivate his conclusion:
For much, and perhaps most, of modern medical practice, RCT-based data are lacking and no RCT is being planned or is likely to be completed to provide evidence for action. This “dark matter” of clinical medicine leaves practitioners with large information gaps for most conditions and increases reliance on past practices and clinical lore. 4,69,70 Elevating RCTs at the expense of other potentially highly valuable sources of data is counterproductive. A better approach is to clarify the health outcome being sought and determine whether existing data are available that can be rigorously and objectively evaluated, independently of or in comparison with data from RCTs, or whether new studies (RCT or otherwise) are needed.
Seriously, try searching the literature on this. It is remarkably consistent on this point. Like, I’m shocked there’s not more disagreement.
For those trying to build intuition as to why the two types of studies seem to have similar evidentiary value over time despite the radically different design, consider the following: RCTs have excellent internal validity: their results—when properly run—are quite likely true for the patients they worked with, at the time and place they were run. But there’s a whole set of murky issues around the question of external validity: how exactly to interpret these results, and what do they mean for the real world. Observational trials may have lower internal validity—given the inherently noisier signal they’re working with— but their external validity is much easier to establish, since they tend to use a lot more patients, and the environments they’re testing are far more realistic than the insulated world of RCTs.
As a result of the above intuition, my sense is that if we want the highest level of confidence, we’re better off looking to evaluate our hypotheses with both observational trials and RCTs, not just the one or the other.
If parallax is good enough to give us 3d vision out of two eyes that can only see in 2d, maybe it’s good enough to help us perceive depth in clinical questions also.
Systematic denigration of observational trials and their removal from the evidence pool doesn’t seem to match what we know, though it’s a common trope on Twitter.
Scott continues:
They found that using the kit led to a 75% or so reduction in hospitalization, though they were unable to separate out the ivermectin from the other things in the kit (paracetamol and aspirin), or from the placebo effect of having a kit and feeling like you had already gotten some treatment (if I understand right, the decision to go to the hospital was left entirely to the patient).
I think this study is a moderate point in favor of giving people kits in order to prevent hospital overcrowding, but I’m not willing to accept that it tells us much about ivermectin in particular.
Is the interpretation being put forward here that giving people kits of placebo medicines is a more plausible explanation for 75% reduction in hospitalization than ivermectin having an effect? Does that pass the smell test?
In the words of a wise person on Twitter:

Look, I understand the “RCTs alone can show us the truth” position. It is intuitive. It is clean. It feels right. It has mathematical elegance. But, we now know that when tested in the real world, well, it’s not true. RCTs don't seem to yield more information than observational studies. One more messy truth to deal with, I suppose.
Mayer et al.
Here’s how Scott describes this one:
Mayer et al: Not an RCT. Patients in an Argentine province were offered the opportunity to try ivermectin; 3266 said yes and become the experimental group, 17966 said no and became the control group.
While we do know that the 3266 were indeed offered the opportunity to try the treatment, we do not know that the remaining 17966 “said no”. It appears that physician discretion was heavily involved:
However, the inclusion of patients was left to the discretion of the treating physician and to the acceptance by the patient. For this reason, the non-inclusion of patients in the IVM program could be due both to the refusal of patients to receive treatment after it was offered, to non-compliance with the inclusion criteria, or to the decision by the treating physician not to offer this treatment option
Moving on:
There were many obvious differences between the groups, but they all seemed to handicap ivermectin. There was a nonsignificant trend toward less hospitalization and significantly less mortality (1.5% vs. 2.1%, p = 0.03).
While looking into this study, I learned the term “immortal time bias”. This means a period in between selection for the study and the beginning of study recording where patient outcomes are not counted. I think the problem here is that if you signed up for the system on Day X, and if you got sick before they could give you ivermectin, you were in the control group. See this Twitter thread, I have not confirmed everything he says.
As far as I can tell, what Scott calls “Immortal Time Bias” is discussed in the paper as “Survivor bias” which is used interchangeably in some of the literature. The paper not only recognizes the issue, it actually mentions that even if they exclude all patients who died within four days of inclusion, the results of the analysis maintain the "statistical significance" of the mortality result in the high-risk subgroup (patients aged over 40).
Survivor bias was assessed and controlled in a sub-analysis (data not shown) through the exclusion from the analysis of all individuals in the control groups whose death occurred within the first 4 days since diagnosis, since those individuals were in all likelihood not offered the intervention, maintaining a significant association in favor of IVM in terms of mortality frequency in the higher risk groups (non-immunized subjects older than 40 year-old).
Given that it was a retrospective study, the complaint about pre-registration in the thread Scott linked is unusual, and the p-hacking concerns seem to imply that subgrouping by age is somehow something strange, which... it isn't.
Keep in mind that the ivermectin group, even after any survivor bias-style reassignment, appeared to be at higher risk (~2x as likely to have hypertension and ~3x as likely to be obese), as Scott noted. In fact, every single risk factor seemed higher in the ivermectin group over the control group. This might reflect physician bias to encourage high-risk patients to join the treatment or something else.

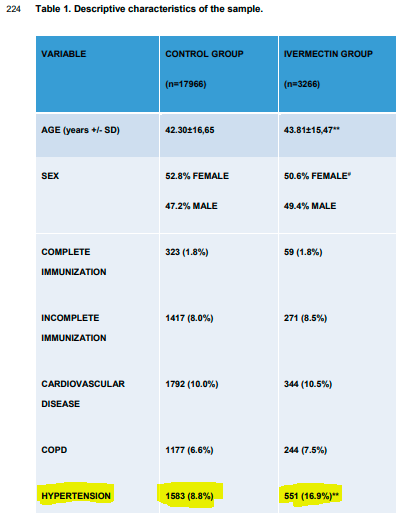
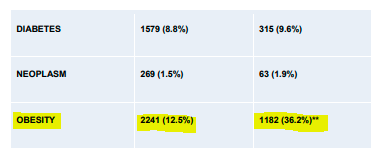

I suppose we should call this “anti-survivor bias,” since the ivermectin patients were clearly at more risk, for whatever reason. From the paper, we can see that both hypertension and obesity led to significantly higher levels of ICU admission and mortality.
Still, somehow, the treatment arm came out significantly ahead.
This is another one of those cases where maybe there is some criticism to make of a trial (there always is) but the strength of the result can’t simply be explained away by the criticism levied.
Scott concludes:
This only hardens my resolve to stay away from non-RCTs.
Look, I get it. Observational trials are uncomfortable to work with because they’ve got all these statistical issues to reason through. We need to balance the pro-treatment factors with the anti-treatment factors and decide if we feel the difference can explain the results.
However—and this is crucial—RCTs simply brush the uncertainty issue under the rug. Instead of understanding those issues as they arise within the study, we have to consider them later, to weigh the study’s applicability in the real world.
Pot-ay-to, Pot-ah-to. There ain’t no such thing as a free 🥔.
Chahla et al.
Scott writes:
Chahla et al: The first of many Argentine trials. 110 patients received medium-dose ivermectin; 144 were kept as a control (no placebo). This was “cluster randomized”, which means they randomize different health centers to either give the experimental drug or not. This is worse than regular randomization, because there could be differences between these health centers (eg one might have better doctors who otherwise give better treatment, one might be in the poor part of town and have sicker patients, etc). They checked to see if there were any differences between the groups, and it sure looks like there were (the experimental group had twice as many obese people as the controls), but as per them, these differences were not statistically significant. Note that if this did make a difference, it would presumably make ivermectin look worse, not better.
The primary outcome was given as “increase discharge from outpatient care with COVID-19 mild disease”. This favored the treatment; only 2/110 patients in the ivermectin group failed to be discharged, compared to 20 patients in the control group.
But, uh, these were at different medical centers. Can’t different medical centers just have different discharge policies? One discharges you as soon as you seem to be getting better, the other waits to really make sure? This is an utterly crap endpoint to do a cluster randomized controlled trial on.
So, are we going with the hypothesis that patients in the control group were 7.6 times more likely to still be hospitalized by day 5-9 because of different discharge criteria in the different centers? Isn’t it more likely that—since this trial was run by Ministry of Health researchers—the centers used centrally-determined criteria?
The clinicaltrials.gov registration gives a little more detail about what went into discharge:
Medical release: numbers of participants with absence of clinical symptoms relation to COVID-19 disease.
Of course, I do understand that “absence of clinical symptoms” still requires medical judgement, but nothing that would explain the divergence we see in the results.
What’s more, the secondary outcome is “reduction in percentage of participants with symptoms (PPS).” Given that it, too, gives us a similar result to the primary, that acts as disconfirmation of the hypothesis that discharge criteria were behind the observed difference.
If you’re going to do cRCT, which is never a great idea, you should be using some extremely objective endpoint that doctors and clinic administrators can’t possibly affect, like viral load according to some third-party laboratory, using the same third-party laboratory for both clinics.
Once more, the “RCT Über Alles” mentality does not seem to be borne out by the data. While this is a less well-studied question, the analyses I can find don’t seem to find much difference between cluster RCTs and traditional RCTs:
For binary outcomes, CRTs and IRTs can safely be pooled in MAs [meta-analyses] because of an absence of systematic differences between effect estimates. For continuous outcomes, the results were less clear although accounting for trial sample sizes led to a non-significant difference.
Naturally, the paper has to invent new terminology, so what they call CRT is a Cluster RCT, and what they call IRT (Individually Randomized Trial) is what we all know as an RCT. Anyway, you get the point.
This is such a bad idea that I can’t help worrying I’m missing or misunderstanding something. If not, this is dumb and bad and should be ignored.
As discussed, I think Scott’s concern isn’t something that can explain the results we’re seeing, especially given that the secondary outcome, which doesn’t depend on discharge policy, is also pointing in the same direction.
Conclusion
In this essay, we see three studies Scott snubs mostly because they’re not the “gold standard” RCTs. I hope I demonstrated sufficiently that not only is there no justification for why such studies should be discarded, but that including them in a meta-analysis in addition to RCTs helps diversify the sources of evidence, giving us a more robust foundation for conclusions. While reasonable people may disagree in the case of cluster RCTs (and Chahla et al. in particular), the case for including large observational trials seems pretty straightforward.
Appendix: The Removal of Merino et al.
I want to make sure I cover a specific element here that Scott didn’t mention in his essay, because someone will likely bring it up in the comments. Merino et al. has been removed from the preprint server that hosted it. This story should probably be its own article, but until then, I’ll try to give the highest-level timeline summary of events I can:
The Merino et al. study was published on SocArXiv on May 4, 2021.
In August 2021, Politifact went after the study with an argument boiling down to “Merck said ivermectin doesn’t work, so how could it work?” and “What is causality, really?” Slight strawman, but only slight.
By December 2021, SocArXiv threw the study under the bus, calling it “debunked” (based on Politifact?!) but kept it on the server.
Then JP Pardo-Guerra went after the study on Twitter, with a thread accusing the authors of undeclared conflicts of interest, and—I kid you not—comparing it to the Tuskegee experiment:

The authors were pretty clear about working for the Mexico City government, and they were clear that the program they were writing about was also run by the Mexico City government, so “undeclared conflicts” appears to be an odd accusation.
Accusing a local government of using a drug that other countries and/or the WHO don’t believe works shows a pretty poor understanding of how national sovereignty functions.
Of course, none of this mattered. In February of 2022, SocArXiv buckled, removing a paper from the server for the first time in its history. The gist of the retraction:
To summarize, there remains insufficient evidence that ivermectin is effective in treating COVID-19; the study is of minimal scientific value at best; the paper is part of an unethical program by the government of Mexico City to dispense hundreds of thousands of doses of an inappropriate medication to people who were sick with COVID-19, which possibly continues to the present; the authors of the paper have promoted it as evidence that their medical intervention is effective.
What is incredible is that they come within an inch of saying out loud that the reason for the retraction is that they don’t like the implications of the result.
When the director of SocArXiv was asked why the authors weren’t given an opportunity to respond, he answered:
The lead author responded on Twitter, not that it made any difference:
Sobre la decisión de @familyunequal de eliminar del sitio que dirige el análisis sobre Ivermectina y Covid en la CDMX. Le enviamos la siguiente respuesta. drive.google.com/file/d/1VyRIFV… Agradecemos su lectura y divulgación. Muchas graciasThings got more complicated when it turned out one of the original authors was pleased with the removal…
…and then it turned out that the lead author had fired him a few months earlier:
From there it was off to the races. RetractionWatch, Washington Post, Mexican Press all jumped on the story, recycling the talking points.
Of all the critiques of the study I’ve seen, nothing comes close to retraction-worthy, never mind the first-ever removal from a preprint server. But of course, we’ve seen worse.
None of that stopped Gideon Meyerowitz-Katz, writing in the BMJ, from framing the study as if some rogue administrator surreptitiously tested a drug on hundreds of thousands of patients just to write a paper about it.
This requires a full article to explore it thoroughly, but I wanted to park my research notes here until then, so anyone concerned has the links to work through, at least.
This is a public peer review of Scott Alexander’s essay on ivermectin, of which this is the ninth part. You can find an index containing all the articles in this series here.
In addition:
I'm surprised how much Scott Alexander distorts Merino's study when he describes it. As if he hadn't read it.
SA's claim 1: "if you called a hotline and said you had COVID, they sent you an emergency kit".
That is not what the study says. Patients who received a positive test for a test were *given* a kit (under certain conditions.) SA seems to be making a confusion with the phone *monitoring* a subset of the patients were offered: they were called a few days after they had received a positive test, to check how they felt and to advise them to go to hospital according to the description they gave of their own health state.
SA's claim 2: "18,074 people got the kit"
Inaccurate : 83,000 patients got hte kit, out of whom 77,381 were included in the study. Among whom 18,074 received a monitoring phone call.
SA's claim 3: "Their control group is people from before they started giving out the kits, people from after they stopped giving out the kits, and people who didn’t want the kits."
Where does that come from? Mexico didn't stop giving out the kits (not right after the study, at least). Here's what the study mentions: "The control group are positive symptomatic patients, from 23 November to 28 December, and the treated group are positive symptomatic patients from 28 December to 28 January."
(The City of Mexico started delivering the kits to any person who tested positive — under certain conditions — as from Dec 28th 2020)
SA's claim 4: "There are differences in who got COVID early in the epidemic vs. later, and in people who did opt for medical kits vs. didn’t. To correct these, the researchers tried to adjust for confounders"
The reasons the authors adjusted for confounders are not exactly the ones SA mentions. Unlike SA is suggesting, there is no "early in the pandemic" involved in the study : the time difference between control group and treated group is exactly 1 month: Nov 23-Dec 28 2020 for the control group, and Dec 28 2020-Jan 28 2021 for the treated group.
Besides — and this has nothing to do with Scott Alexander — thanks to your paper, I've just found out that Merino's paper has been withdrawn by the preprint server where it had been published. And the reasons are incredible: https://socopen.org/2022/02/04/on-withdrawing-ivermectin-and-the-odds-of-hospitalization-due-to-covid-19-by-merino-et-al/
> But, we now know that when tested in the real world, well, it’s not true. RCTs don't seem to yield more information than observational studies.
There are famous counter-examples like hormone replacement therapy: https://archive.ph/lCCwf
Cochrane does try to integrate non-RCTs but with care: https://training.cochrane.org/handbook/current/chapter-14#section-14-2