This is a public peer review of Scott Alexander’s essay on ivermectin, of which this is the seventh part. You can find an index containing all the articles in this series here.
For this installment, I’ll focus on how Scott addresses (or doesn’t) the twin issues of trial design and statistical power.
When a scientist approaches an instrument, be it telescope or microscope, the first question is one of the capabilities of the instrument itself, as well as its calibration. We don’t look at a sample through an out-of-focus microscope and conclude that cells do not exist, for instance. Conversely, 100 failed measurements due to ill-calibrated equipment don’t outweigh the evidence of a few well-calibrated measurements. Scientists tend to understand that observations outside of everyday phenomena require a lot of precision, where every part of the inferential chain must be correctly aligned. Getting any important detail wrong can lead to missing a real signal.
At the top of his essay, Scott indicates that he does have some familiarity with the issue at hand:
“doesn’t affect to a statistically significant degree” doesn’t mean it doesn’t work. It might just mean your study is too small for a real and important effect to achieve statistical significance. That’s why people do meta-analyses to combine studies.
And yet, as Scott reviews the ivermectin literature, the issue of whether each trial he looks into is properly calibrated so that its results can be considered meaningful, doesn’t even enter the conversation.
There are many ways to underpower a trial. Instead of just walking through Scott’s reviews of various studies and pointing out errors, I’ll take this opportunity to use examples to illustrate a few of the many ways in which a trial can fail to reach “statistical significance,” even if the result is there.
Too Few Patients: Mohan et al.
Scott writes:
Mohan et al: India. RCT. 40 patients got low-dose ivermectin, 40 high-dose ivermectin, and 45 placebo. Primary outcomes were time to negative PCR, and viral load on day 5. In the results, they seem to have reinterpreted “time to negative PCR” as the subtly different “percent with negative PCR on some specific day”. High-dose ivermectin did best (47.5% negative on day 5) and placebo worst (31% negative), but it was insignificant (p = 0.3). There was no difference in viral load. All groups took about the same amount of time for symptoms to resolve. More placebo patients had failed to recover by the end of the study (6) than ivermectin patients (2), but this didn’t reach statistical significance (p = 0.4).
Overall a well-done, boring, negative study, although ivermectin proponents will correctly point out that, like basically every other study we have looked at, the trend was in favor of ivermectin and this could potentially end up looking impressive in a meta-analysis
If one reads this text, the takeaway from Mohan is that ivermectin probably doesn’t work: “…it was insignificant (p = 0.3),” “…didn’t reach statistical significance (p = 0.4),” “…a well-done, boring, negative study.”
The first thing that jumps out is Scott’s confusion of “statistically non-significant result” with “negative study.” Trials can have positive results without reaching statistical significance, and this is especially true when the design of the trial is such that reaching statistical significance requires implausible levels of effectiveness.
Reading all this, I wondered about the calibration of the study. Was it adequately powered? One way to figure this out is to see how the study decided the number of patients it needed to recruit. The investigators usually state a hypothesis that sounds something like this:
“Patients like the ones we will recruit get hospitalized x% of the time. If we expect the drug to have y% effectiveness against hospitalization, and we want 80% certainty that our study will identify that effect reaching statistical significance, we need z patients in each arm.” This still requires several assumptions—and assumes every measurement is fully precise—but at least it shows some kind of thinking going into setting the size of the study to balance a reasonable budget with the highest practical opportunity to statistically capture the intended effect, if it exists.
Instead, if we read the paper on the Mohan et al. trial, we see the following:
You just can’t make this up. But I did use a screenshot, just in case someone thinks I did. They’re literally saying the quiet part out loud: they used as many patients as they could be bothered to find, “as this was a pilot trial of a repurposed drug in a pandemic setting.” What does being a repurposed drug have to do with anything, other than to demonstrate that orphan drugs are most easily abused by medical science? How did peer review allow this through? How in the world can we call a study “null” or even “negative” (never mind “well-run”) when there was no consideration given to whether there were enough patients recruited to reach “statistical significance,” even in principle?
That sentence is the equivalent of using an out-of-focus telescope to prove that the Andromeda galaxy does not exist. If I make a study with five patients on whether water helps with dehydration, and the result is that “4/5 patients were cured, but water failed to reach statistical significance,” would Scott call it a “negative study” and advise people to stop drinking water? I assume not.
The Mohan et al. study is a perfect example of a case where the concept of statistical significance is worse than meaningless: it’s harmful. Anyone who describes this study as negative (or “null”) is exposing themselves to well-deserved criticism.
Too Many Cycles: Chaccour et al.
Chaccour et al: 24 patients in Spain were randomized to receive either medium-dose ivermectin or placebo. The primary outcome was percent of patients with negative PCR at day 7; secondary outcomes were viral load and symptoms. The primary endpoint ended up being kind of a wash - everyone still PCR positive by day 7 so it was impossible to compare groups.
First, Scott says that “everyone was PCR positive by day 7.” This isn’t right: one of the ivermectin patients was actually negative. This is fairly minor, but it will become very relevant shortly.
Reading about the study setup, I got curious about what exactly “PCR positive” meant in this context. As we found out from our investigation into the Biber study, the cycle threshold can make all the difference. Weirdly, the paper never specifies the number of cycles used as a threshold in declaring a patient negative, but it does offer a few clues:
The sample size was based in the comparison of two proportions and calculated to have 80% power at a 5% significance level to detect a 50% reduction (100 vs 50%) in the proportion of participants with positive PCR at day 7 post-treatment. The 100% PCR positivity figure at day 7 is based on the experience with COVID-19 outpatients at the Clínica Universidad de Navarra during the first wave of March-May 2020
In other words, 100% of patients in the first wave of March-May 2020 tested PCR positive on day 7. Does this sound like a PCR test that might be overly sensitive? And why would they expect ivermectin (a single dose, even) would be able to clear half the patients by day 7? The authors don’t offer any rationale for that assumption. Remember that PCR tests don’t only pick up live virus, but also RNA fragments— essentially viral debris that the body takes a while to clear out. As a result, depending on PCR test sensitivity, coming up negative by day 7 may be all but impossible. For all we know, the ivermectin patient that tested negative on day 7 might have been their first patient to ever do that. Looking at the paper, something stood out:
In the diagram above, by day 7, if we try to find the cycle threshold where just one ivermectin patient is negative, that would be 40 cycles. I have also confirmed this by looking into the study dataset itself, which is available for download online (kudos for releasing it!). Nowhere in the paper or the appendices is this noted. I have to assume this is because it would stand out like a sore thumb. You see, above 33-35 cycles, you’re almost guaranteed to be picking up viral debris only. CDC today uses 28 cycles as the threshold for positivity, which has about even odds of being live virus or debris:
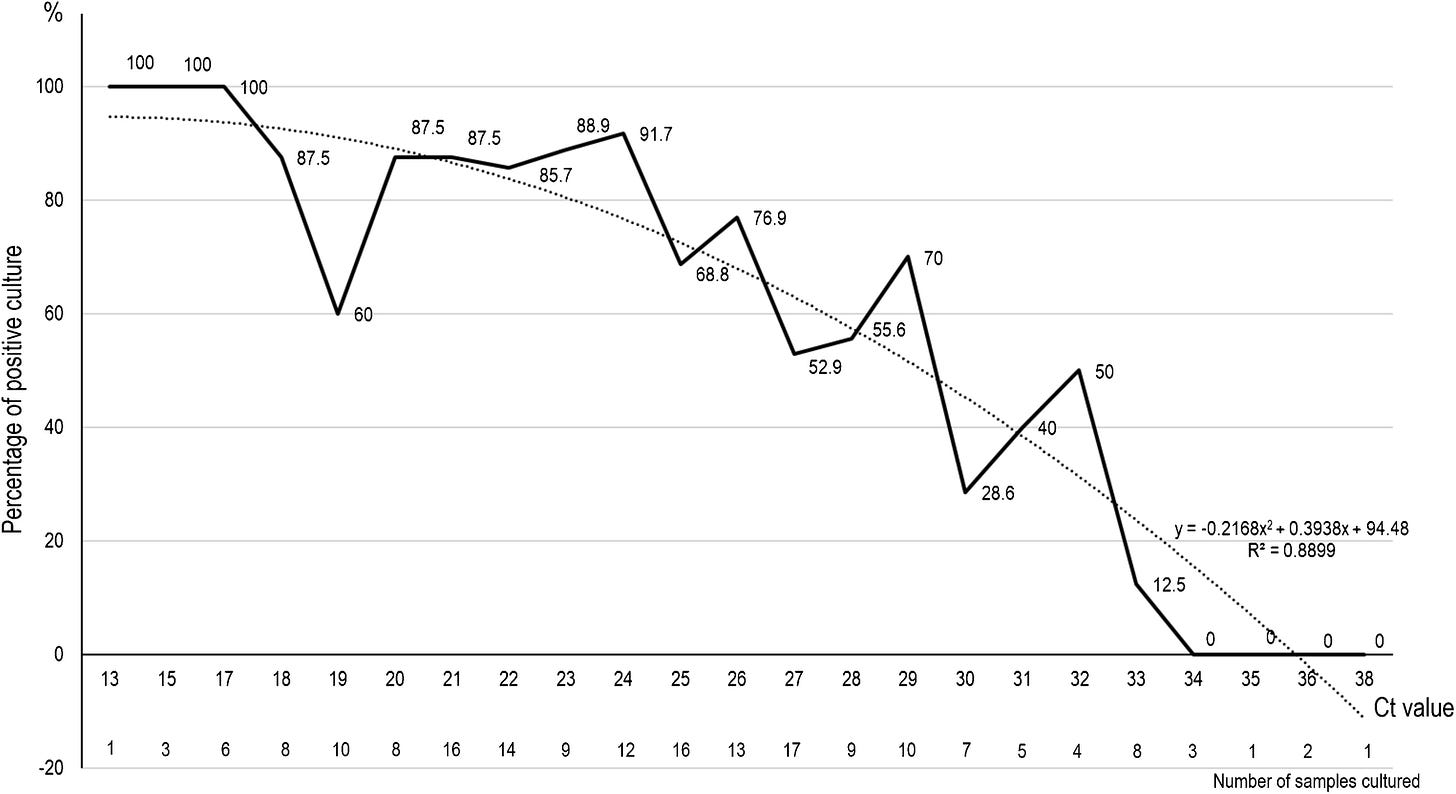
In the words of one Anthony Fauci, at the time the Chaccour et al. trial was starting:
If you get a cycle threshold of 35 or more, the chances of it being replication competent are miniscule. Somebody comes in and they repeat their PCR and it’s a 37-cycle threshold, but you almost never can culture virus from a 37-threshold cycle. So, I think if somebody does come in with 37, 38, even 36, you gotta [sic] say it’s just dead nucleotides, period.
Turns out that the cycle threshold used for PCR tests is yet another way to calibrate (or miscalibrate) your study when PCR positivity is your endpoint. Chaccour did not pre-declare 40 cycles as his threshold in the pre-published protocol, and there is no explanation as to why that was the threshold chosen.
In fact, in what the authors make sure to tell us is “post-hoc” analysis, they actually did find that when you set PCR cycle threshold to 30 or less, you get statistically significant results for one portion of the PCR test (gene E), and borderline significance for the other (gene N). It’s unclear why the results are being split (it wasn’t in the pre-registration). ivmmeta says that when you average the two, the result is statistically significant.
If we use the released patient data to recompute the primary endpoint results with the 30 cycle threshold (the same as what was used in Biber et al.), we get something that looks completely different than the published results:
ivermectin group, day 7: positive 3, negative 9
placebo group, day 7: positive 8, negative 4
Scott continues:
Ivermectin trended toward lower viral load but never reached significance.
Here’s what the first author has to say about the viral load data:

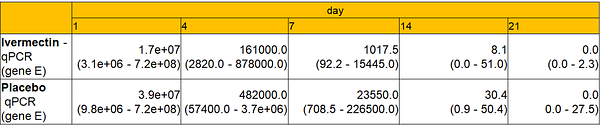
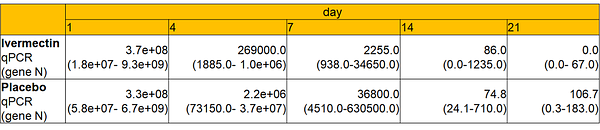
If that was not enough for it to reach “significance,” what is the point of designating p=0.05 as a threshold that has any meaning whatsoever in this context?
@sudokuvariante over on Twitter has a great illustration of the issue. I’ve resized his charts so they’re on the same scale, and placed them side-by-side below. On the left, you can see the log10-transformed viral load reduction relative to baseline in the Hammond et al. Paxlovid trial. On the right, you can see the same, for ivermectin, in the Chaccour trial.
So why is the Paxlovid result considered “statistically significant” and the ivermectin result not?

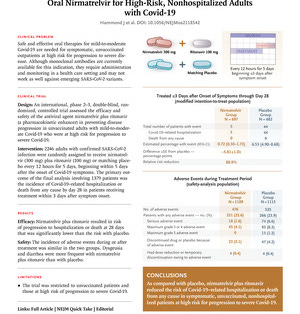
I hope the issue of trial calibration and statistical power is starting to come through by now.
Let’s keep going:
Weirdly, ivermectin did seem to help symptoms, but only anosmia and cough towards the end (p = 0.03), which you would usually think of as lingering post-COVID problems.
Weirdly? Given the remarkable reduction in viral load? Scott makes an effort to pin anosmia as a “post-COVID problem” but the data from the trial (figure S5) makes it abundantly clear that that’s not the case. More than half the patients report it from day 0. If it lingers, it is because it doesn’t get treated.
Let’s keep going though.
The paper says:
Given these findings, consideration could be given to alternative mechanisms of action different from a direct antiviral effect. One alternative explanation might be a positive allosteric modulation of the nicotinic acetylcholine receptor caused by ivermectin and leading to a downregulation of the ACE-2 receptor and viral entry into the cells of the respiratory epithelium and olfactory bulb. Another mechanism through which ivermectin might influence the reversal of anosmia is by inhibiting the activation of pro-inflammatory pathways in the olfactory epithelium. Inflammation of the olfactory mucosa is thought to play a key role in the development of anosmia in SARS-CoV-2 infection
This seems kind of hedge-y.
It does, doesn’t it? Especially given that the writeup is indistinguishable from an attempt to bury a real signal of efficacy. Scott continues:
If you’re wondering where things went from there, Dr. Chaccour is now a passionate anti-ivermectin activist:
Ironically, Chaccour is sharing an article from the BBC which makes wild claims about the findings of the “fraud hunter” squad that Scott has based much of his article on. In this article, they claim that researchers not sharing their data is a red flag for fraud. The irony? The “fraud hunters” have not shared the data they used to make those wild claims of widespread fraud in the ivermectin literature in the BBC article, even though they promised me to release them “soon.” At the very least, this should give you a sense of how circular the anti-ivermectin media frenzy is.
Let’s continue:
Long-term consequences (***to poor, rural people in developing countries***) of the misuse of ivermectin data. The old story of actions having consequences that stretch beyond the perpetrators.So I guess he must think of this trial as basically negative, although realistically it’s 24 people and we shouldn’t put too much weight on it either way.
Yup. A “basically negative” trial with order-of-magnitude reduction of viral load, statistically significant reduction in anosmia/hyposmia, and when PCR cycle threshold is set to a reasonable 30 cycles (instead of an unreasonable 40 cycles) we also see:
9 of 12 patients were PCR negative in the ivermectin group vs 4 of 12 patients being PCR negative in the placebo group
Statistically significant difference in viral load progression
It’s almost like Dr. Carlos Chaccour didn’t become a passionate anti-ivermectin activist; it’s almost as if he was motivated to show a certain result regardless of the data. Now, why would one do that? A quick Google search turns up something:
Carlos serves as the Chief Scientific Officer of the Unitaid-funded BOHEMIA project
So what’s the BOHEMIA project?
I generally try to stay away from questioning people’s motives and funding sources, but for UNITAID, I’ll make an exception. Chaccour being listed as Principal Investigator of a $25MM UNITAID-funded project is relevant information.
You see, that’s the same organization that Dr. Andrew Hill is on record (and on video) admitting that they explicitly asked him to commit academic misconduct by modifying the conclusion of his early and wildly positive ivermectin meta-analysis, by adding text specifically designed to delay a positive recommendation by organizations such as the WHO. One of the most stunning, smoking-gun admissions of the whole pandemic. Here’s the video:

(If you prefer to read a transcript, it can be found here.)
Well, this took a strange turn towards the conspiratorial. Let’s get back on track, shall we?
Too Small a Dose: Ahmed et al.
Scott writes:
Ahmed et al: And we’re back in Bangladesh. 72 hospital patients were randomized to one of three arms: ivermectin only, ivermectin + doxycycline, and placebo. Primary endpoint was time to negative PCR, which was 9.7 days for ivermectin only and 12.7 days for placebo (p = 0.03). Other endpoints including duration of hospitalization (9.6 days ivermectin vs. 9.7 days placebo, not significant). This looks pretty good for ivermectin and does not have any signs of fraud or methodological problems.
Fantastic! Or?
If I wanted to pick at it anyway, I would point out that the ivermectin + doxycycline group didn’t really differ from placebo, and that if you average out both ivermectin groups (with and without doxycycline) it looks like the difference would not be significant. I had previously committed to considering only ivermectin alone in trials that had multiple ivermectin groups, so I’m not going to do this.
Scott seems to be missing something pretty important about this trial.
The three arms are:
1. Ivermectin (12mg x 5 days)
2. Ivermectin (12mg x 1 day + Doxycycline)
3. Placebo
Scott has not even mentioned the fact that the one arm is delivering five times the dose of the other. If anything, the pattern of the results is evidence of a dose-response relationship. Instead, it appears that Scott thinks that any amount of ivermectin is as good as any other. Underdosing is a common way of underpowering a trial, and that’s why phase 2 studies often try multiple doses to see if one works better than another, before moving to phase 3.
But there’s something else that’s strange about what Scott claims.
if you average out both ivermectin groups (with and without doxycycline) it looks like the difference would not be significant.
I have no idea why Scott would think that “averaging out” both groups would be the right way to pool the results. I’m no stats guru, but as far as I can tell, when you pool two groups of patients into a single group, the new confidence interval you get is not the average of the constituent groups. If it were, we’d do meta-analyses by a simple average—and we most certainly don’t.
If anyone with a stronger statistics background can help steelman and/or check Scott’s assertion against the paper itself, please leave a comment. I’d be more than happy to update this section with a more precise evaluation of the claim.
I can’t find any evidence this trial was preregistered so I don’t know whether they waited to see what would come out positive and then made that their primary endpoint, but virological clearance is a pretty normal primary endpoint and this isn’t that suspicious.
The pre-registration issue is fair, though the authors do mention they got ethics approval, which indicates they had a protocol before the study started. But it would indeed be useful to see it.
It’s impossible to find any useful commentary on this study because Elgazzar (the guy who ran the most famous fraudulent ivermectin study) had the first name Ahmed, everyone is talking about Elgazzar all the time, and this overwhelms Google whenever I try to search for Ahmed et al.
For now I’ll just keep this as a mildly positive and mildly plausible virological clearance result, in the context of no effect on hospitalization length or most symptoms.
Not much to comment on this, so I’ll just sum up the section: ignoring the dosing of a study is a sure-fire way to get confused about what the results mean. Regardless, combining arms after the fact is a form of meta-analysis, and I’m pretty sure that Scott didn’t actually do one. If he did, he would show us the results and not call it “averaging.” For both of these reasons, casting doubt on this study on the basis of a passing impression is not really fair, but it does betray either a misreading of the study or a misunderstanding of the importance of dosing, given the extreme dose divergence between the arms.
Too High Efficacy Targets: Buonfrate et al.
Scott writes:
Buonfrate et al: An Italian RCT. Patients were randomized into low-dose ivermectin (32), placebo (29), or high-dose ivermectin (32).
It should be noted that what is called a low-dose arm in this context is dosing at 0.6 mg/kg for five days, 2.5 times the dose that the TOGETHER trial called “high-dose,” which was 0.4 mg/kg for three days. The high-dose arm in Buonfrate dosed at 1.2mg/kg for five days, a whopping five times more than the high-dose arm of the TOGETHER trial. A trial dosing this high should really produce a result, if adequately powered. Let’s see what happened, shall we?
Primary outcome was viral load on day 7. There was no significant difference (average of 2 in ivermectin groups, 2.2 in placebo group).
There is so much to say about just this last sentence. First of all, the paper reads:
The reduction (expressed in log10) was 2.9 for the higher dose (arm C), 2.5 for the lower dose (arm B) and 2.0 for placebo
So Scott gets the numbers completely wrong. I can’t find where the number 2.0 is coming from for ivermectin, or where 2.2 is coming from for placebo.
Worse still, he ignores the fact that the paper is expressing its results in log10. So the placebo arm had a viral load reduction of 100x, the low-dose arm had a reduction of ~312x, whereas the high-dose arm had a reduction of ~794x. That means that the high-dose arm reduced viral load by eight times as much as placebo, and the low-dose arm had a reduction of three times as much as placebo. It should be noted that this kind of gradient is also consistent with a dose-response effect, with higher doses having a more pronounced effect.
Even if his numbers were right, Scott criticized Krolewiecki et al. for “subgroup slicing that you are not supposed to do without pre-registering it,” yet here he’s happy to do “subgroup merging” that the authors didn’t do and didn’t intend to do. There’s no nice way to say this: I get a sense that Scott has no appreciation for dosing whatsoever, something that anyone opining on antiviral studies really should care more about.
That’s a lot of substantial errors for a single sentence, isn’t it? But he’s right that the result was not “statistically significant.” Scott elaborates in his next sentence:
They admit that they failed to reach the planned sample size, […]
Oh.
[…] but did a calculation to show that even if they had, the trial could not have returned a positive result.
Oh? Here’s what the authors actually wrote:
However, the conditional power (CP) analysis showed that even reaching the target sample size, the hypothesized effect would hardly be demonstrated (arm B vs. arm A, CP = 0.001; arm C vs. arm A, CP = 0.27).
Compare Scott writing definitively that “the trial could not have returned a positive result,” to the authors stating that the high-dose arm had 27% chance of reaching “statistical significance,” given the current data.
I have no idea how Scott read this and interpreted it as “the trial could not have returned a positive result.” Also note how smoothly Scott replaces “statistically significant” with “positive,” as if no distinction exists between the two terms.
Scott closes thus:
Clinically, an average of 2 patients were hospitalized in each of the ivermectin arms, compared to 0 in the placebo arm - which bucks our previously-very-constant pro-ivermectin trend.
Strangely enough, Scott—who tends to zero in on systemic differences between populations—does not mention the significant differences between groups here, with three times as many patients in each of the ivermectin arms having the baseline visit in a hospital setting, and the high-dose arm having large differences in baseline gender (73% vs. 45%), higher baseline cough (56% vs 42%), pyrexia (56% vs 33%), and anosmia (33% vs 17%). All these indicate that the two ivermectin arms may have received patients who were more ill, especially the high-dose arm. Adding to that suspicion is that the patients in the high-dose arm also had higher baseline viral loads on average. Given that Scott and the “fraud hunters,” on whose research he relies, tend to jump on these sorts of “systemic imbalances” for pro-ivermectin papers, you’d expect this to be noted.
Was Buonfrate Sufficiently Powered?
Let’s leave all the errors aside for a moment, though. Let’s assume Scott had correctly conveyed the findings of the paper. Does the study add or subtract evidentiary weight to the hypothesis that ivermectin, administered in adequate dosing and at the right time helps with COVID-19?
What jumped out at me, is that the study observed roughly 3x and 8x reductions in viral loads relative to placebo in the two ivermectin groups, and yet the result did not reach the threshold of significance. I dug into how they determined the number of patients they needed for the study so that their conclusions are meaningful—what is called the statistical power calculation. The paper says:
The desired difference in decrease between each experimental group and control was at least 0.47 log10 copies/mL (effect size Δ = 0.68, considered to be of moderate-large magnitude according to Cohen [20]). According to these hypotheses, with 34 participants per arm the study had a power of 80% to detect a difference between control and experimental arms, at 0.025 α level, one-sided.
To unpack this, with 34 patients in each arm, the authors estimated an 80% chance of finding a difference in log viral load that had a Cohen’s d value of 0.68 or greater, or somewhere between moderate and large according to the terms Cohen used.
To unpack this further, what a Cohen’s d of 0.68 difference in log viral loads actually means—according to my calculations—is that the ivermectin arms would have needed to demonstrate something like more than 18x reduction in viral load for the result to reach “statistical significance.”
In fact, the high-dose arm reached a Cohen’s d of 0.48, which is “only” a 7.9 times reduction in viral load, on average.
This may sound like the study made unreasonable demands, but given that viral loads can rise exponentially, perhaps this is more reasonable than it sounds?
Let’s look at other similar studies on pharma-backed treatments, to see what they look for and how they calibrate their investigations.
How did BLAZE-1 approach this question?
Simon Vallée on Twitter suggested looking into BLAZE-1, the study of bamlanivimab-etesevimab, one of the monoclonal antibodies deployed to fight COVID-19:

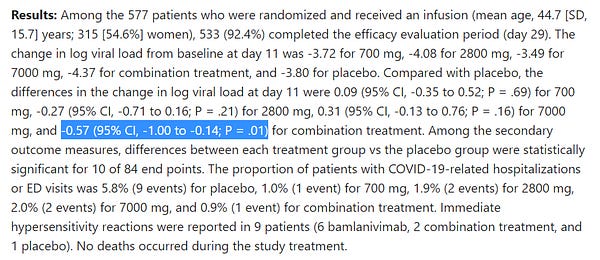
This sounded pretty damning, so I wanted to dig deeper into what that study found, and what action was taken as a result of it.
First and foremost, Simon’s evaluation matches mine. While the high-dose ivermectin arm in Buonfrate et al. got an effect of Cohen’s d=0.48—which didn’t reach the limit of 0.68 in order to be found significant—this study of monoclonal antibody bamlanivimab-etesevimab tried four separate arms, the best of which (combination treatment) got to a Cohen’s d of 0.15.
Cohen's d = (2.43 - 2.16) ⁄ 1.80 = 0.15
On the basis of the results shown here, bamlanivimab-etesevimab proceeded to phase 3, which—after optimizing on the basis of phase 2—found that:
The mean reduction in the viral load from baseline to day 7 was approximately 16 times as high in patients who received bamlanivimab plus etesevimab as in those who received placebo
In other words, the optimized phase 3 study still would not have reached the >18x reduction threshold required by Buonfrate to reach significance. And yet, the combined phase 2/3 study was the basis for the FDA granting an EUA to bamlanivimab-etesevimab.
So why did a smaller effect size become statistically significant for bamlanivimab-etesevimab when a greater effect did not rise to the level of significance for ivermectin? Simple: the ivermectin study was so small that it could only reach significance if the effect was larger than the monoclonal antibody demonstrated. The bamlanivimab-etesevimab phase 2 study included 577 patients, more than 100 patients in each arm, and 156 in placebo. Compare this to Buonfrate et al. with 30 or fewer patients in each arm.
Given the size of the study, anything other than an earth-shattering effect was bound to be found “not statistically significant,” and then rounded down by commentators to a “negative” study showing that ivermectin “doesn’t work.” And yet, by the time the study was cut short, the conditional power analysis concluded that there was still a 27% probability that the high-dose arm would reach significance—against the near-impossible target—if only some more patients were recruited to the study.
One More Comparison: Molnupiravir
In the Merck phase 2a study for Molnupiravir, the following chart appears:
I’ll spare you the squinting, here’s the raw data for day 7, so we can compare with Buonfrate:
Before we compare results, note that the Molnupiravir study used “least squares mean,” which is a way to improve precision by using various statistical adjustments. Buonfrate did not do that, using a naive mean.
Still, we see that the highest dose of Molnupiravir had a log viral load reduction difference of -0.534. In Buonfrate, the low dose of ivermectin had a reduction difference of -0.5. The high dose did much better, with a reduction difference of -0.9. Both studies even have a similarly small number of participants.
And yet, the resulting writeup on viral load reduction for Molnupiravir—in contrast to what Buonfrate wrote for ivermectin—is glowing:
Conclusion
In evaluating clinical trials, context is king. The most common criticism that ivermectin proponents have towards the various “non-significant/negative/null” trials is that they are statistically underpowered. This could be due to insufficient number of patients, dose, waiting too long to administer treatment, using low-risk patients, etc.
At the same time, the criticism that comes back is that no trial seems satisfactory and that proponents are asking for an impossible trial. Instead, as we’ve seen here, a trial that is equally well-calibrated as the trials for other early treatments—the ones backed by large pharmaceutical companies—would likely show ivermectin to be just as effective, if not more. Things being as they are, we should approach the ivermectin trial set, and in particular the various statistically underpowered studies, as fragments of evidence whose conclusions have little if any weight on their own, especially when interpreted within a frequentist framework. Better use of evidence is, of course, possible, but we’d need to convince people to look into different statistical frameworks, and that fight is even bigger than this one, so it will have to wait.
Here, I’ve shown that Scott not only fumbles numbers and routinely conflates “statistically significant” with “positive” and “statistically non-significant” with “negative,” he also accepts at face value what the various researchers claim—absent criticism from the one-sided stable of experts he used for his piece—even though he’s perfectly happy to be hyper-critical of other researchers where their findings conflict with the direction he’s headed towards. As a result, he misses the rich and varied ways in which a trial can be underpowered, confusing a weak signal for a “negative” result.
If you can’t imagine your favorite early treatment (monoclonals, Paxlovid, Molnupiravir, fluvoxamine…) producing a statistically significant result under the trial conditions and analysis framework ivermectin has been put under in a given study, chances are ivermectin’s results will also be lackluster.
Perhaps part of the problem is that trial leads are assumed to be doing their best to ensure that their trials are appropriately powered and calibrated to find an effect, if one exists. However, as we have seen, when the intervention being tested is repurposed, off-patent, and/or generic—and the investigators have no skin in the game, especially when politics enters the set of considerations—that cannot be taken for granted.
When evaluating evidence, it’s important to examine the instrument used for a measurement—and whether it’s even capable of discerning an effect—before treating its results as affirming the hypothesis of no efficacy.
Well, that was quite a journey, eh? As always, please leave any corrections or omissions in the comments.
This is a public peer review of Scott Alexander’s essay on ivermectin, of which this is the seventh part. You can find an index containing all the articles in this series here.
THANK YOU for tackling Scott's smear piece on ivermectin, Alexandros. I only learned about it myself when a commenter at The Burning Platform version (https://www.theburningplatform.com/2022/07/24/letter-to-alex-berenson-on-world-ivermectin-day/comment-page-1/) of my "Letter to Alex Berenson on World Ivermectin Day" (https://margaretannaalice.substack.com/p/letter-to-alex-berenson-on-world) shared a link to Scott's article, stating, "Deep research assessment by Scott Alexander which suggests the author of this piece has her head firmly up her ass. Her credibility just dropped to nil."
Also, I wanted to let you know the link you have for part two says it is private, so I cannot view the article. I would like to share this series in response to that commenter but want to get the correct link first. Thanks, Alexandros!
I generally don't like talking about motives either; but I agree calling out unitaid is the right thing to do. Likely the deafening silence will continue, and there is little legal basis for taking action against them, if any. But if I was science czar id seek to have their organization permanently blacklisted from my country. Not out of concern for IVM; but out of a general concern for scientific ethics. People talk a lot about the potential for money to have implicit influences on science; but I do not recall ever hearing of something so brazen as this affair involving unitaid. The way things are going, people are going to start thinking that you disclose your funding sources not for an implicit risk of bias, but that funding sources literally dictating conclusions is infact 'the new normal'.